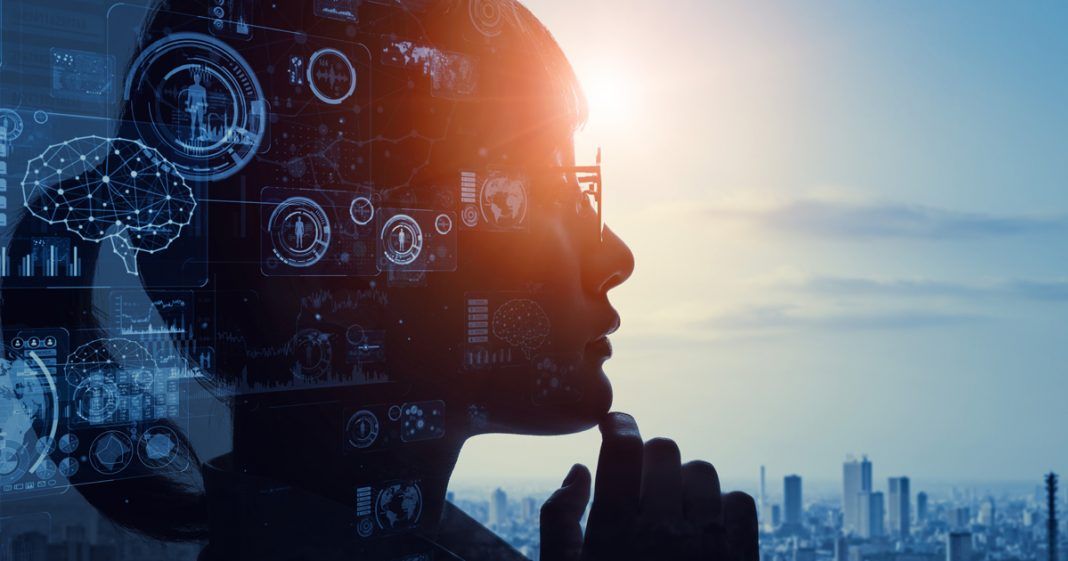
The importance of data management in the successful deployment of data science and machine learning (ML) cannot be overemphasized. Of course, data is that elephant in the room that no one wants to talk about. It’s that gnarly beast that keeps data scientists, citizen data scientists, and data analysts up at night. But the integrity of the data that you use in processing your advanced analytics is crucial to achieving the results that every business is looking for: those pivotal insights that could potentially be game-changing.
In a new best practices report, Data Management for Advanced Analytics, 2020, research firm Transforming Data with Intelligence helps shed some light on how to get data management right for one of the hottest trends in advanced analytics today–machine learning.
According to the report, “Machine Learning and AutoML are only as good as the data fed to them. Here’s the catch: the newfound abilities of machine learning and AutoML depend heavily on getting the right data at the right time to the correct models” (TDWI, Data Management for Advanced Analytics, 2020, Philip Russom).
In the report, Russom lays out the five cycles of machine learning and the different, yet equally demanding data requirements for each cycle. The cycles that Russom alludes to in machine learning are:
- Solution definition
- Development
- Deployment
- Production
- Monitoring output
To make the content more easily digestible and understandable, Russom provides a diagram to illustrate the different data requirements by stage:
As you can see, ensuring that machine learning models have the right data at the right time is a complex task. Use this report to make sure you satisfy all the requirements of each stage of machine learning – the future of your business may depend on it.