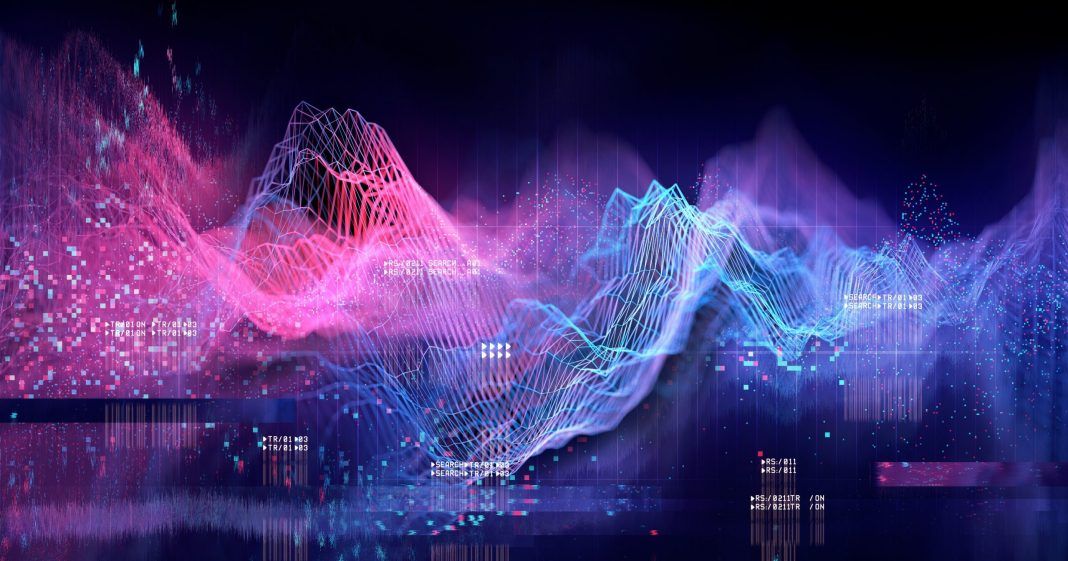
Successful master data management (MDM) is crucial to the success of daily operations, analytics, and compliance efforts. But many organizations forget about some key facets of master data, leading to poor MDM strategy and lackluster business performance. That’s why we’re publishing this blog series, highlighting commonly overlooked facets of MDM.
In this post, we will examine the problem of modeling. We’ll focus on data modeling, saving workflow modeling for an upcoming post on governance and lifecycle management.
At its core, the data model is documentation. For an MDM project to be successful, you need to be clear on what you will be governing. And that means you need to document, in an unambiguous way, the values and the relationships you plan on managing with your MDM.
This raises many questions, the most important of which we’ll cover below.
- Can I use someone else’s model? It depends on what you’re mastering. In cases where you’re mastering fairly simple things like standard code lists, country/postal codes, securities symbols, you can use someone else’s model. But most firms are looking to manage more complex entities, such as financial data, organizational hierarchies, customers, products, and locations-data that are specific to their organization. The more specific the need the less relevant a third model becomes. At some point, the costs of learning, analyzing, and customizing someone else’s model outweigh the benefits of starting with a vendor-built, or industry-standard model.
- How can I deal with different data models patterns? One of the difficulties of master data modeling is the wide range of model patterns. In most cases, you will think of a relational model with “flat” tables, but as you look at the data and its relationships you’ll notice that you’ll need to define other types of structures. Some data models are highly hierarchical, with recursive relationships between nodes. Other data models must contain dynamic structures. If your MDM solution cannot absorb all those different patterns, there is a risk that you might implement an overly rigid model. That lack of flexibility translates into hard coding a lot of behaviors and rules on top of a physical model, increasing the cost of implementation and making any change expensive and time consuming.
- How can I define business rules to enforce validation? Usually, the development of an application starts with the design of a data model, and then the coding of business rules and processes. In MDM, the challenge is that the business rules that enforce validation must be part of the data model. From simple control like min/max values to complex algorithms with functions and operators, your business rules are part of the definition of your master data. To achieve flexibility in the MDM, it is necessary that rules are linked to the data model definition and rely on the same life cycle.
- How will I deal with models’ lifecycles? Whether or not you choose to use an external tool or your MDM platform’s built-in modeling feature, how you will manage multiple versions of your model over time is an important consideration. MDM is all about the governance of your master data. While people immediately focus on the values and relationships, you should also remember what you choose to govern may expand and change over time, especially if you are moving from managing a single domain to a much broader, multi-domain implementation. This expansion in scope translates into a change in the model and necessitates a means of maintaining model versions.
- How will I integrate my models into the MDM platform? Standards-based integration always looks great on paper, but vendors have subtly different interpretations of the standard. This means if you are planning on using an external tool, be prepared to perform moderate processing work to clean up your model after importing it into the MDM platform. In theory, built-in modeling tools don’t suffer from this integration problem. But this depends on what “built-in” really means. Some vendors “built-in” modeling by grafting multiple products together. The hodgepodge works about as well as you would expect (generally, not so well).
- Can I use the models for more than documentation? While the purpose of your model is to document what you are choosing to govern, the purpose of your MDM project is to define, execute, and maintain a governance protocol for this information. As documentation, your model has significant value. It provides a common platform for achieving consensus between all interested parties and guides the development and maintenance processes. But what if you could do more than just read the documentation? This is an area where MDM platforms that are model-driven stand apart. Model-driven MDM platforms use their models as direct input into the development and maintenance processes. This also means that you can take a more agile, collaborative approach in designing your MDM platform.
In this post, we discussed a few of the main elements of modeling that we think many people miss in the early days of their MDM program. For more on key facets of your master data, read this comprehensive guide on the six business-critical facets of master data that organizations often overlook.
And keep a lookout for our next post in which we cover issues related to relationships when describing different data model patterns.