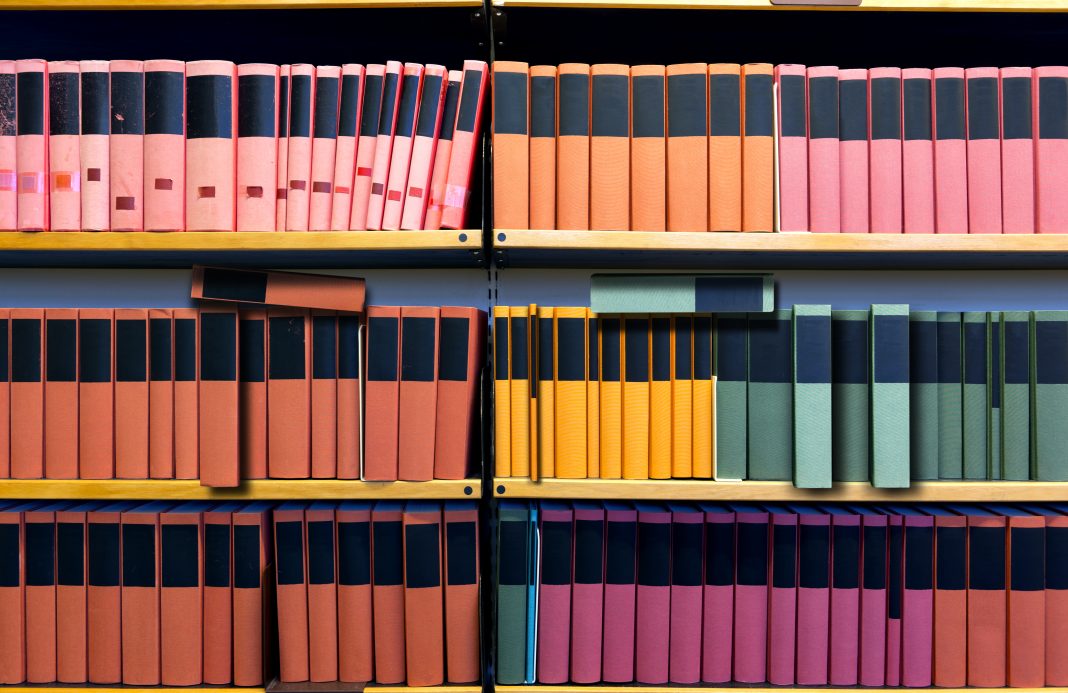
Data lakes are a great idea. They must be; there sure are a lot of them these days!
Here are some reasons why they are proliferating:
- Different applications: Examples might be an analytic data store for data scientists, another a repository for offloading cold data, and a third, low-cost storage and compute in the cloud.
- Workload distribution: If query and analytical workloads grow too large, splitting lakes into multiple, possibly overlapping data lakes can spread the workload.
- Decentralized teams: Different divisions or departments may inadvertently set up separate data lakes.
- Geographically distributed users: If data science teams are spread across the globe, a centralized data lake may result in unacceptable network delay.
- Platform consolidation: With so much change in the big data vendor landscape, organizations may temporarily have duplicate lakes as they migrate towards a common standard.
- Regulations: Data regulations may prohibit the storage of certain types of data together in one data platform, and in some countries, regulations prohibit data from leaving the country.
- Mergers and Acquisitions: Acquiring another company adds the acquisition’s data lakes to the acquirer’s mix.
Silos, silos, and more silos
Since there seems to be a myriad of good reasons for each data lake, one might ask, what’s the downside?
The downside is that by adding multiple, distributed data lakes to their already vast array ofdata sources, enterprises now have more data silos than ever before. And each new silo makes it just a little bit harder for businesses to access, mine, analyze, and derive value from their data.
Addressing the “Now we have even more silos” challenge
So, what should enterprises do? In a recent webinar, Rick van der Lans, a highly-respected independent analyst specializing in data warehousing, business intelligence, big data, database technology, and data virtualization identified three ways that enterprises can fuse together their distributed data lakes and other important data sources. The options he outlined were:
- Integration by data science tool
- Integration by data replication
- Integration by data virtualization
For each of these options, Rick compared their various implementation aspects, such as
So, which option does he recommend?
I would love to tell you, but you’ll have to find out yourself. To gain an in-depth
By the way, all webinar participants will also receive a copy of Rick’s technical white paper on this topic, “The Fusion of Distributed Data Lakes: Developing Modern Data Lakes.” So, don’t miss out.