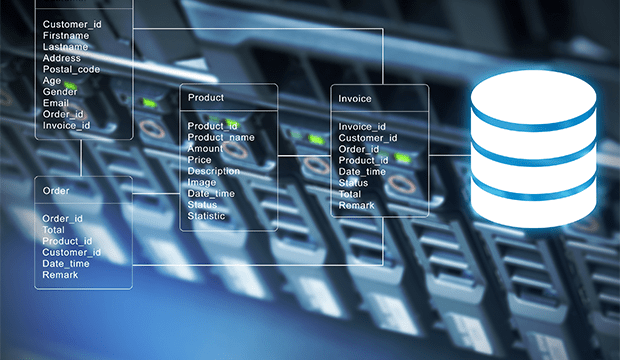
This is a follow-up blog post in our Graph Database series. Learn what a graph database is and why it’s important here.
Today’s approach to fraud detection: Discrete Analysis
With its terrific ability to enable users to spot patterns quickly and easily, graph database has wide implications for use in a number of industries including fraud detection. Fraud accounts for billions of dollars lost per year and it seems each year, fraudsters get more sophisticated in outsmarting the banks. Unfortunately, the most common attribute of fraud is misdirection and burying their patterns in lots of data. With traditional data storage techniques, it really isn’t possible to see beyond individual points to the connections between them. That sort of heightened, overarching pattern-like view has now become more feasible with graph database. The connected data view in graph database can more easily uncover these larger complex patterns and make fraud evasion harder. To understand how useful an addition graph database can be in detecting fraud, it’s best to first understand Gartner’s layered approach to fraud detection.
Gartner analysts say “No single layer of fraud prevention or authentication is enough to keep determined fraudsters out of enterprise systems.” They say that you need a combination of monitoring and controls to combat fraud. Therefore, they recommend following the 5 layered fraud prevention approach. This approach recommends that companies use all five layers, ranging from the most basic of security measures (secure browsing) to the most complex (analysis of relationships), and encourages companies to face the fact that some determined fraudsters will break through the first few layers of security. Because some will make it in.
Most of today’s available fraud prevention solutions only address the first four layers of Gartner’s recommended approach. For instance, banks mainly use transaction monitoring systems (TMS) which rely on relational databases. Due to their linear, discrete analysis approach, most of today’s solutions can usually only spot trends and incidents after they’ve happened and only a limited basis.
While the discrete approach is an easy one that helps users spot patterns and capture fraudsters acting alone, it doesn’t necessarily detect patterns between all the different data endpoints and therefore, is not very useful in detecting elaborate crime rings. Further, modern fraud rings have become very familiar with the ways of discrete data analysis and know how to avoid detection with this approach. This leads us to the next frontier of fraud detection which revolves around connected analysis.
Tomorrow’s approach to fraud detection: Connected Analysis
This is where graph database can really add value. Graph database addresses Gartner’s fifth layer of fraud prevention: entity link analysis. Graph database enables banks to look beyond the individual data points of discrete analysis to the connections that link them. With graph database, banks can see their data in “graphs” and more easily visualize patterns and opportunities to better predict when and where fraud might occur.
Another important trait that makes graph database a value add to any fraud prevention solution is its inherent speed in calculating relationships. Since the relationships in graph database are treated with as much value as the database records themselves, the engine that navigates the connections between nodes can do so efficiently, enabling millions of connections per second. Graph database enables quick extraction of new insight from large and complex databases to help uncover unknown interactions and relationships. This means that with a graph database, banks can process data and compute risks quicker than today’s current relational databases so they can spot opportunities and threats before the competition.
According to Forbes, graph database reduces false positives, improve false negative detection, ease investigations, and reduce overall fraud investigation costs. Fundamentally, fraud detection depends on the ability to analyze the relationships between customers and transactions, and recognize patterns or trends. All at the speed of today’s transactions. Graph database provides the speed and the ability to detect large patterns making it the ideal addition to any fraud prevention solution.