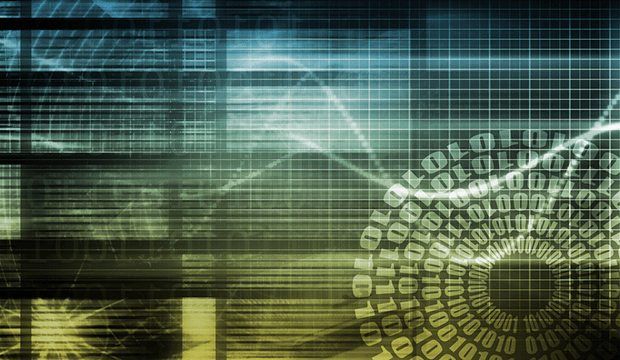
The volume of new data being generated around the world is growing exponentially. Indeed, the digital universe is doubling every two years and is expected to reach 44 zettabytes or 44 trillion gigabytes by 2020, according to IDC. Moreover, IDC predicts enterprise storage compound annual growth rates of more than 50 percent through 2016.
As the volume of data continues to expand, so too do the costs for companies to gather, cleanse, and store data—even as storage technologies continue to become more efficient and the cost per gigabyte of hard disk storage continues to fall.
As spending on data continues to rise, decision-makers will need to devote greater attention in determining which data sets are providing the greatest operational or financial returns—and those that aren’t. These practices will likely become more prevalent as there’s more widespread recognition of data as an organizational asset that is valued and measured.
Data valuation will apply both to companies that “productize” their data and sell it in one form or another (e.g. Google, Yahoo, Moody’s), as well as consumer packaged goods (CPG) manufacturers, retailers, banks, utilities, travel/hospitality, and companies in other industries that are able to obtain insights and draw value from data.
While there are certain data types that companies are legally required to retain for specific periods of time in industries such as financial services and healthcare, there are other types of customer, business, and market data that lose shelf value after a prolonged period of time. According to the Data Warehousing Institute, 2 percent of records in a customer file becomes obsolete each month due to death, bankruptcy, and relocation.
Visual and predictive analytics software can help decision-makers to identify and remove unneeded data from being stored, as well as outdated data that’s no longer valid or useful. For instance, IT professionals for a CPG company can use analytics to identify out-of-date inventory data. Retail managers can examine POS data to determine whether it makes sense to retain information on customers who haven’t made purchases for years. Manufacturers can review sensor data from equipment to assess the utility of historical data in predicting leading indicators of failure conditions.
Bottom line is that not all data is of the same value in running a business. Customers with repeat purchases have more information than those that don’t when it comes to assessing product affinity. Sensor data showing (failure) events have more information than sensor data collected while machines are running without incident, for anomaly detection.
In our modern world of Fast Data, one needs to: (a) identify leading indicators of business-valuable events (e.g. purchases of products, failures of equipment), (b) back-test these leading indicators against historical data, and (c) monitor the steady stream of ongoing Fast Data to make an informed intervention (e.g. an offer to a customer or a service on equipment). Smart companies are able to save the most informative data to enable rapid and accurate identification of such leading indicators. This data enables valuable interventions with customers, machines, and assets to robustly grow the business.