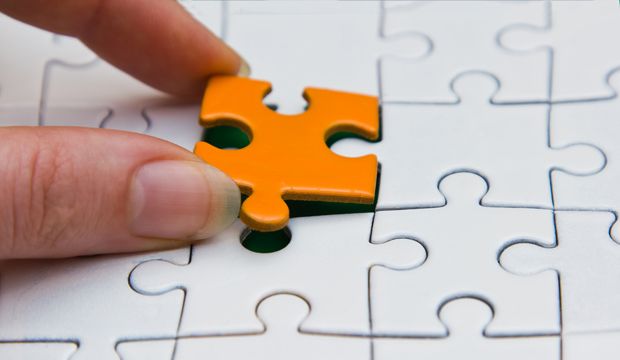
Sometimes, just sometimes, what happens in Las Vegas shouldn’t stay in Las Vegas. That was clearly the case this week when TIBCO CTO Matt Quinn took the stage to talk about the myths and realities of Big Data. In Why Big Data Won’t Make You Smart, Rich or Pretty, Quinn provided his perspective on Big Data based on years of experience in some of the biggest data environments around, like FedEx, Nielsen, and others.
Forget the ‘big’ part for a moment; think about variety.
Quinn pointed out that most customers are not struggling with ‘big’ data but are instead still struggling with data. In Quinn’s view, it is the complex interactions between customer data sets that cause the majority of the issues. Success depends more on piecing together different data sets across wildly different applications and systems, with variety of data being the key.
In Quinn’s opinion, solving this data ‘jigsaw puzzle’ is often overlooked and tools like Hadoop, while clearly in focus, is just one tool in the toolbelt and can be a clumsy tool when dealing with real-life complexity.
New “new” application architecture
Quinn went on to say that he’s seeing more and more focus given to aspects of Big Data and Cloud as people build new business applications. According to Quinn, these applications have common elements that stand out:
- Operational data is still being stored in a regular relational DB
- MongoDB, Cassandra, Giga, ActiveSpaces used more frequently to handle transient and fast-moving data for real-time analysis
- With direct integration to some flavor of Hadoop for offline or batch-oriented analysis
The resulting analysis is still being stored in an operational data store as of today, but that’s mostly due to the various web framework support available, more than anything else. This will change as new frameworks gain support, leading to an increase in use of the new architecture.
The Big Momentum Challenges
Early iterations of Big Data projects were driven as science experiments, often managed by consultants long on theories but short on practical experience. This drove vendor overload and left the marketplace without many specific success stories for a particular industry or a particular problem. For some, it became an excuse for IT to ‘play around’ with data. Unfortunately, getting a handle on the real challenge means taking a step backward to think about the data itself more than its size. It’s hard to take that step backward for most organizations as it involves decisions, investments and personalities.
Obstacles and Opportunities
Quinn raised data security and organizational change as two of the biggest obstacles and opportunities. Societies and our various cultures are being affected by the privacy and other questions that Big Data raises, leading to data sovereignty and protection legislation entering the discussion. Getting specific, Quinn talked about PCI data and the need to anonymize or keep ‘chinese walls’ between business units that could abuse the public’s or customer’s trust with too much access.
On the issue of organizational change, Quinn points to the science experiments that are not helping to inform companies of what potential change looks like. Before the Big Data cycle passes, there will need to be serious thought about data governance and data stewardship, and how that gets set up and managed. Quinn painted the following scenario to emphasize his point:
- Imagine you have thousands of applications and databases
- Each has an owner and (probably) a specific business domain and is often from a specific/unique perspective
- Each is guarded by various IT and business gatekeepers
- Trying to piece together this data set – in a consistent manner – often takes a centralized data governance group
- And in most cases these groups have failed (re: company-wide data warehouse initiatives)
These are very real issues that others beyond Quinn are starting to raise.
What does it matter if you can’t act on it?
Toward the end, Quinn put up a graph that highlights the business value of data as it decays over time as a way to point out that insights are most valuable when the capture, analysis and decision latency can be kept to a minimum. His customers that are getting the highest value from data aren’t necessarily looking for needles in haystacks, but instead are engaged in shortening the cycles that cause data to lose value.
This point stresses the aspect of big data that is most often overlooked… that finding, understanding and deciding how to act on data may very well mean more than amassing more data and taking longer to get to the place where the organization can move.
From a debunking of the hype point of view, Matt Quinn’s talk was a great start to a day focused on myths and realities of Big Data.