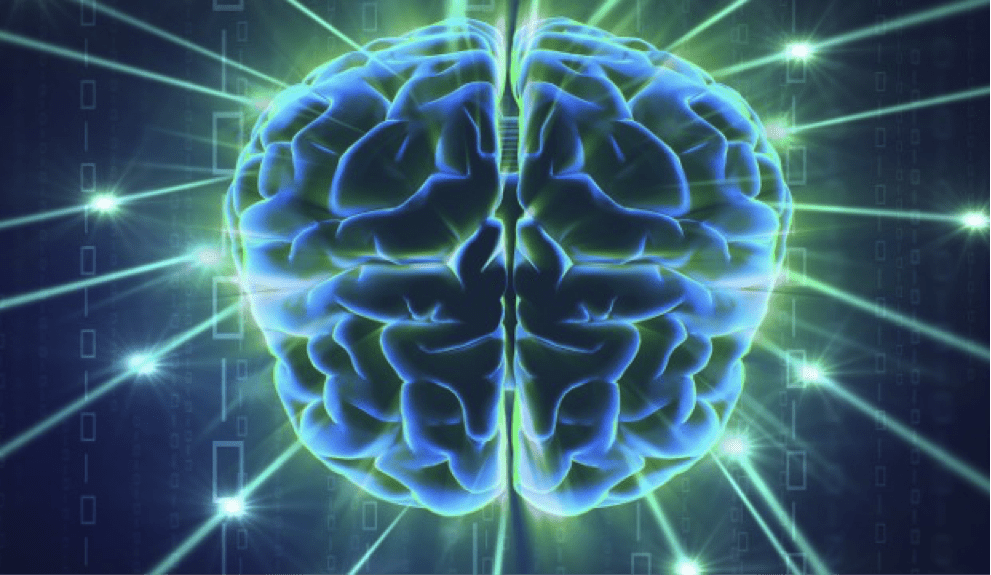
Machine learning is being heralded as the next leap forward in the data analytics and business intelligence (BI) space. Although machine learning has been around since the 1960s, recent advancements with new algorithms are enabling computers to dramatically improve analytical output over time as machine-learning algorithms gain experience.
Such improvements are making it possible for marketers to determine the most effective offers to extend to prospective customers and for network analysts to detect and respond to potentially fraudulent activity faster and more accurately.
In order for machine learning to work in use cases when time is of the essence, in-the-moment data about customer behaviors and operating conditions are critical for making instantaneous assessments between historical and current data.
For instance, companies often monitor social media buzz for sentiments that are shared about brands. Real-time data analysis, combined with machine learning, can be used to alert company leaders about a potentially harmful product problem that’s gaining traction on social media before it goes viral, including recommendations on the most effective steps to take to address the problem.
Likewise, machine learning that draws upon Fast Data can help a retailer to analyze how a customer is responding to a mobile advertisement, and what the next best action the company should take to engage that customer to help drive conversion (e.g. share a video about that product based on the response rates of customers with similar characteristics).
One of the most powerful applications for machine learning is detecting unauthorized network intrusions. Machine learning algorithms can help network analysts detect intrusions using real-time streaming data, and when combined with the use of a Fast Data platform, can also correlate and contextualize information to determine whether a network intrusion represents a legitimate threat that needs immediate attention.
Another popular application for machine learning is identifying and predicting equipment failures before they occur, based on current operating conditions of machinery, including temperature and vibration readings, blended with historical operating data. Plant operators need access to real-time operating insights quickly in order to respond before breakdowns occur and to conduct maintenance rapidly without impacting production.