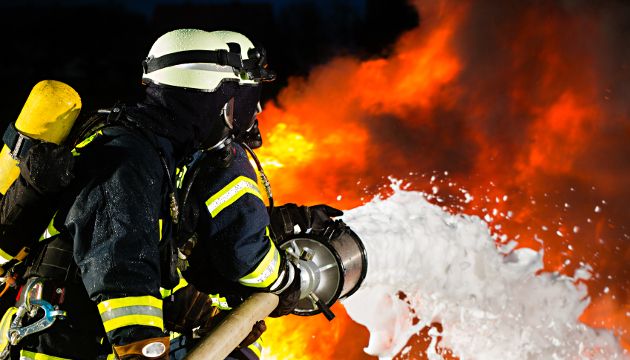
No industry today can shy away from the influences and impact brought on by Big Data. Better yet, there is mass acknowledgment that Big Data’s worth is realized only as much as the analytical insights derived from it to make decisions and take action. One such case: the New York City Fire Department (FDNY) is leveraging Big Data analytics to empower its firefighters to make them more effective and efficient in their jobs.
FDNY is responsible for inspecting 330,000 of the one million buildings in New York City (NYC). Each of their fire companies must perform nine hours of building inspections each week. However, emergency response calls frequently cut into those times, which means that building inspections need to be prioritized so that those with the greatest fire risks can be addressed first. Certain buildings like older structures, buildings with active tax liens, buildings with foreclosure proceedings, and complaints are expectedly at greater risk for fires.
Over a year ago, FDNY deployed a data analytics algorithm, named FireCast 2.0, that helps the fire department manage inspected buildings and prioritize them based on fire risk. Later this year, FDNY plans to launch FireCast 3.0—a new version that will use data collected from the NYC’s non-emergency 311 reporting service and its 17 city agencies, and apply 7,500 risk factors including building specifications, noise complaints, and trash violations, to determine the likelihood of fires.
According to StateTech, the FireCast 3.0 algorithm analyzes three years of data for every building in the city, every night. FireCast 3.0 will then use this data to create a fire risk score based on complex statistical analysis. The buildings are then ranked for inspections and assigned to New York’s 341 fire companies.
“With this new model, we’re creating a risk profile for every battalion in the city. When you begin to chart the buildings within an area, you see how risk scores change over time. There’s generally a strand of buildings that remain risky all the time, and then another strand that remain relatively un-risky. But for those middle buildings, behavioral cues or violation activity turn them on and off in terms of riskiness,” according to UrbanOmnibus.com.
This is a great example of how Big Data analytics deliver tremendous value—in New York, it is preventing fires and saving lives every day.
Next Steps:
- Try Spotfire and start discovering meaningful insights in your own data.
- Subscribe to our blog to stay up to date on the latest insights and trends in big data and big data analytics.