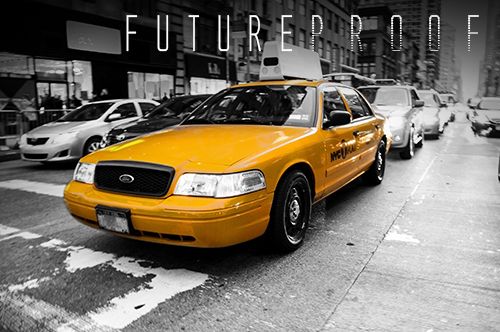
The next step beyond big data is Fast Data. While the use of big data is essential to discover deep insights in infinitely detailed information, the physics of the Hadoop architecture is designed to process data at rest. By contrast, Fast Data is designed to process data while it’s in motion. Fast Data requires a variety of processing techniques. Chief among them are rules-based and stream-based event processing.
Stream- vs. Event-Based Processing
What is the difference between rules-based and stream-based Fast Data processing? And can they be used together? Imagine applying event processing to a moment in the life of a virtual taxi company. We have hundreds of cars and hundreds of customers looking for rides in real time. Let’s examine how Fast Data can help one customer, Nick, get a ride.
In the video below, we show a real-time view of our network. Cars and passengers are tracked in real time, and this real-time command and control application provides a real-time view of our car network. It allows rules and actions to be executed based on real-time insight.
The first element of this Fast Data architecture is streaming analytics. Streaming analytics taps into streams of GPS data from cars, continuously aggregates that data, and joins it in real time with the positional information of customers who, in this case, is Nick. With every move of every car, and each move Nick makes, streaming analytics calculate which cars are closest to Nick based on any selection criteria he can choose (cost, rating, favorites, etc.). This kind of computation cannot be done with traditional big data technology like Hadoop—calculations must be made in milliseconds and constantly transmitted to the drivers, riders, and the company. So streaming analytics is designed to calculate real-time analytics against massive streams of constantly changing data. That’s step one.
From our virtual taxi company’s point of view, how can we optimize which cars are presented to Nick in real time? How do we decide how to price the ride given our knowledge of the available drivers, the customer, traffic patterns, and weather?
Don’t Just Make a Decision, Make the Best Decision
Once we have a continuously streaming real-time view of cars and passengers, event-driven rules can determine the best and most profitable driver for Nick given proximity, history, preference, and desirability. For example, a business rule can determine that Nick has a preference for three drivers that are within an acceptable distance, but that one driver will produce the highest margin and will also likely be the best preference match for Nick given his past riding history. As a result of checking this rule, the company increases Nick’s satisfaction, increases the likelihood of more revenue, and allows our taxi company to present offers and incentives based on rules that were formed from our continuously streaming view of the network.
By combining streaming analytics to continuously calculate a live view of our network, and event-driven rules to continuously check for optimal pairings, our virtual taxi company can now take real-time actions that optimize revenue and customer experience, billions of times a day. This is the meaning of Fast Data; with the proliferation of streaming sensor, GPS, and social data, the need to provide interactive, real-time mobile access to business operations is the future of enterprise computing.