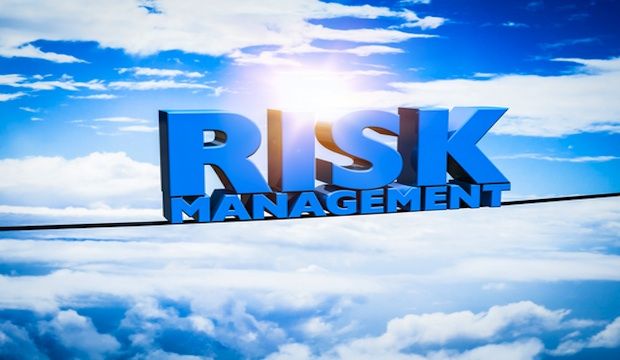
In today’s economy, financial services firms and insurance companies are forced to contend with heightened regulatory environments and a variety of market uncertainties.
That’s why it’s more critical than ever for these enterprises to make effective use of big data analytics to pinpoint and mitigate potential exposure to risk and loss.
The fact is, risk levels remain high and are on the rise for banks and insurance companies around the world.
Many countries that have relatively low risk scores compared to their global peers are seeing their risks increase incrementally, according to a report by Standard & Poor’s Rating Services’ Banking Industry Country Risk Assessment.
In Canada, for example, “the acceleration of household debt to record highs has, in our view, increased Canadian households’ vulnerability to sudden shocks in incomes, employment, or a spike in interest rates,” according to the report.
Since loans by Canadian banks to the consumer sector account for nearly three-fifths of total bank loans, “a substantial shock to household creditworthiness could boost losses on banks’ uninsured loan portfolios,” the report notes.
Meanwhile, insurance companies are also facing an assortment of emerging risks, ranging from the dangers of underwriting property insurance policies in regions that may be vulnerable to Superstorm Sandy-type disasters to the danger associated with underwriting insurance policies for energy companies that are involved in fracking for natural gas deposits.
For their part, insurance companies can apply analytics against a range of customer and market data to gain a deeper understanding of the many factors they face when assessing the risks of underwriting policies for both individuals and businesses.
For example, predictive analytics can help an insurer determine the probability of whether a claim will be applied against a policy that’s issued and how this could impact the long-term profitability of that policy.
The outputs of risk models that are developed by insurance companies are generally more effective “when the model can provide a fully aggregated view of all types of risk,” according to a study by Accenture.
Meanwhile, insurers can also use analytics to better pinpoint instances of insurance fraud, which continues to be a major pain point for the industry.
Questionable auto insurance claims have risen 56% from 2008 to 2012, according to the National Insurance Crime Bureau. A blend of analytics and historical data can help insurers identify and call out questionable claims early in the claims cycle to reduce or even prevent payment on deceptive claims.
Meanwhile, bankers can also reduce risk by applying analytics against a variety of data sets for different types of products.
Many loan officers and underwriters have discovered that an applicant’s credit score alone may not be sufficient enough to determine the risk of default, as a report by Deloitte points out. Bankers can use analytics against macroeconomic, product, and borrower demographic data to more accurately forecast the potential for default before issuing loans.